Artificial intelligence (AI) is widely believed to have a substantial impact on clinical practice. In fact, some areas of medicine have already experienced a substantial growth of AI-based applications, such as radiology where more than 150 FDA or EC cleared products involving AI are available on the market.1,2 This promise is fueled by impressive developments, most recently in particular in the area of large language models that can extract meaning from unstructured text, such as clinical notes, and utilize this information for a broad set of prediction tasks ranging from radiological image annotation to diagnostic classification, the prediction of readmission, length of stay, or comorbidity index.3,4
In psychiatry, the potential promise of novel AI applications is particularly evident. Decades of biological research suggest that biological changes found in affected individuals show typically small effect sizes and are broadly spread across different data modalities.5 For example, a substantial contribution of genetic predisposition to illness risk is evident for most mental illnesses. But the genetic architectures are complex, and the exact mechanisms of how this predisposition interacts with other factors, such as environmental risk, on brain function, is still rather elusive.6 AI has the potential to integrate these small changes into signatures that may be sufficiently predictive to be clinically useful, and to elucidate factors contributing to illness susceptibility at the same time.7,8
Decades of biological research suggest that biological changes found in affected individuals show typically small effect sizes and are broadly spread across different data modalities.5
The application of machine learning to biological data in psychiatry, such as to genetic association, proteomics, or even neuroimaging data, has thus far largely demonstrated that the identification of signatures with a predictive performance sufficient for clinical application, is an enormous challenge. One of the fundamental aspects in this respect is that biomarker discovery studies have thus far large focused on the retrospective analysis of already existing data. The lack of a dedicated study design for biomarker discovery (for relevant considerations, see, e.g.)9,10 complicates the identification of clinically relevant machine learning signatures for several reasons. In particular, such studies were often conducted in a different research context and do not capture the clinical decision challenge that should be supported with the AI application in its entirety. The lack of data for relevant differential diagnostic differentiation of a diagnostic classifier is a typical example of this issue. Furthermore, retrospective data analytics can rarely focus on the classification and prediction tasks that are of direct clinical relevance.
One may argue that recapitulating a diagnostic decision through an AI algorithm is only effective if it also affects therapeutic decision-making, but this may be difficult to assess if the underlying study design is, for example, not longitudinal. Therefore, in order to support the development of clinically useful applications based on AI, there is a significant need for the design of multi-site studies that allow the acquisition of harmonized data resources across time. Such developments are, for example, currently being pursued in a large-scale coordinated effort of the recently established German Center for Mental Health.11
Therefore, in order to support the development of clinically useful applications based on AI, there is a significant need for the design of multi-site studies that allow the acquisition of harmonized data resources across time.
Precision psychiatry through multimodal machine learning
Precision psychiatry has started to move towards multimodal approaches for classification and prediction, since models built on individual data modalities have thus far not yielded the required performance.12,13 The development of such models is becoming increasingly feasible as the acquisition of different data types from the same individuals is becoming common practice (Figure 1). It is important to note, however, that even though such models may be more predictive and more accurately capture the complex illness biology, they may face larger hurdles with respect to their downstream clinical implementation. The increased cost, logistic effort, patient burden, and potentially reduced long-term stability of predictive accuracy, are some of the important considerations.
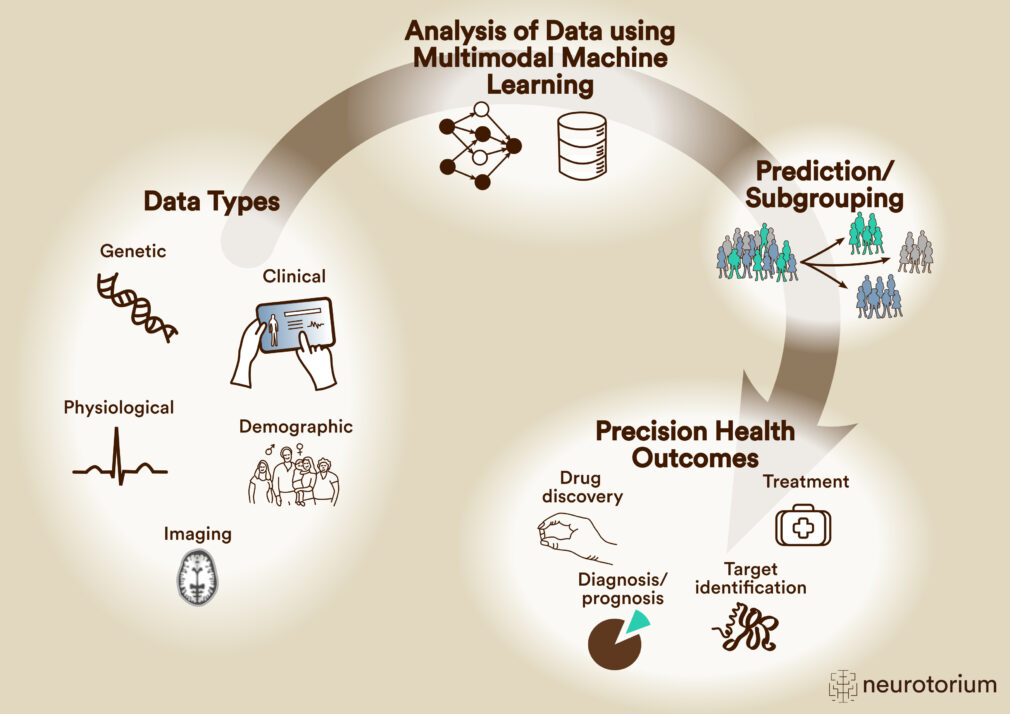
Figure 1: Schematic overview of multimodal machine learning for precision psychiatry
Multimodal machine learning aims to combine different data types in a single predictive model. This may improve the predictive performance of the model if the different data types contribute independent information for a given prediction task. Furthermore, it can give deeper insight into biological mechanisms relevant for illness or therapeutic response. This may guide therapeutic development and facilitate the identification of patient subgroups for which a given illness or treatment-associated signature is of particular relevance.
Advanced artificial intelligence tools and strategies exist, and have started to emerge in research applications, that address this issue, such as learning using “privileged information” or sequential, stacked classifier designs. The former is based on the concept that algorithms are trained on a broader spectrum of data types that are available during the training phase, as compared to the application phase.14 Ideally, such models can profit from the more expansive training data and do not lose too much predictive performance when applied to a reduced test data set. In the latter concept, the stacked models are sequentially built on individual data types, such as clinical, genetic, and neuroimaging information, and the decision to proceed to the next data type is based on the confidence in the prediction of the previous data type.12 This approach has recently demonstrated promising results for the prediction of clinical outcomes in individuals at high-risk for psychosis and in patients with recent onset depression.12
While the prediction of clinical diagnoses has been the core focus of AI applications in psychiatry, it is widely accepted that such assessments may not map well to illness biology.15 Frequently, the biological hallmarks of mental illness are found across diagnostic boundaries, and there is only a minor overlap of biological differences between patients diagnosed with the same condition.16 This large inter-individual heterogeneity suggests that diagnostic constructs do not relate to a patient population with the same underlying illness. However, an intrinsic assumption of most machine learning tools is that this is, in fact, the case, and the algorithms try to identify the signatures that predict these heterogeneous constructs. Therefore, while the models allow prediction at the individual subject level, they are not more personalized than the heterogeneity of the training data allows. Notably, AI approaches can also be used to decipher the heterogeneity between affected individuals, and create a starting point for applications that perform predictions that are more tailored to the individual. Such approaches stratify patients into subgroups that share similar biological and clinical feature values, ideally with respect to the clinical outcome of interest, such as diagnosis or treatment response. This could uncover, for example, that a subgroup of affected individuals with biological changes falling into a specific biological context, may show superior response to a given treatment as compared to the overall population, or other patient subgroups.
Frequently, the biological hallmarks of mental illness are found across diagnostic boundaries, and there is only a minor overlap of biological differences between patients diagnosed with the same condition.16 This large inter-individual heterogeneity suggests that diagnostic constructs do not relate to a patient population with the same underlying illness.
An example where such strategy is being pursued is the COMMITMENT network, which uses machine learning to identify comorbidity signatures of psychosis and cardiometabolic illness, to explore whether such signatures are predictive of metabolic adverse events during treatment with antipsychotics.17 Another interesting example for approaches suitable for stratification that is popular in psychiatric research, but has not found its way into clinical application yet, is that of the so-called “normative modeling”.18,19 Normative models are built on large reference populations to capture the typical development of physiological parameters (such as of brain structure) across the lifespan (Figure 2).20
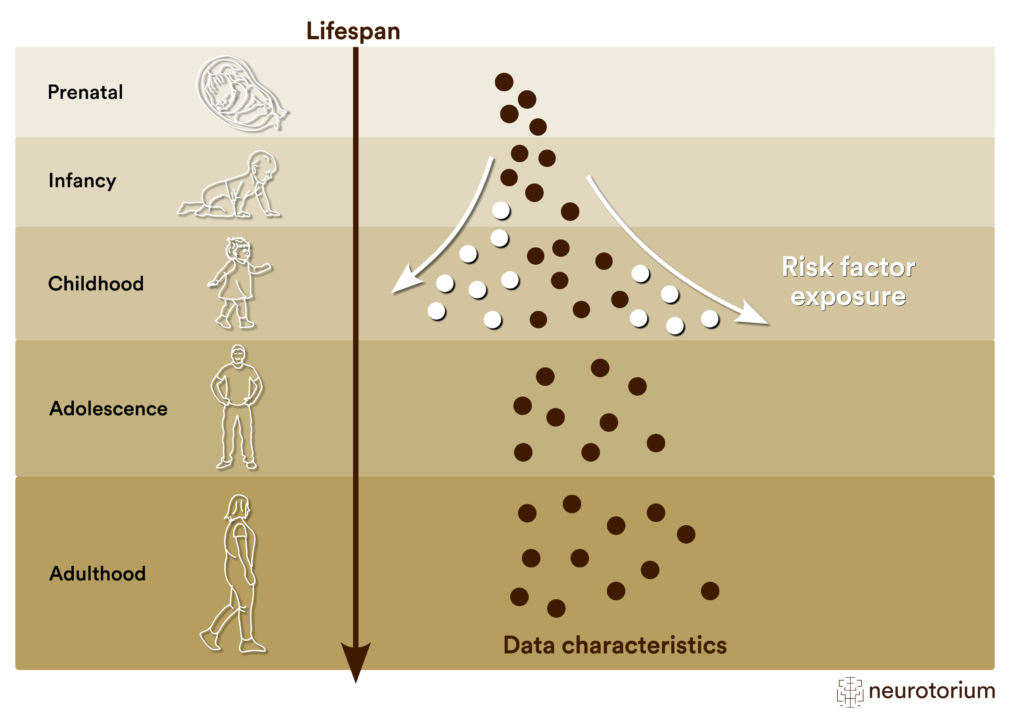
Figure 2: Enabling precision psychiatry by modeling illness mechanisms across the lifespan
The so-called ‘normative modeling’ can be used to model the expected development of a given characteristic across the lifespan. Deviations from this development can be tested for associations with clinical outcomes and may pinpoint illness-relevant effects at the individual subject level. By integrating multiple data modalities this approach may yield information on risk architectures that can be useful for personalized psychiatry.
Based on these models the deviation of a given individual at a particular age can be determined and tested for association with clinical phenotypes. Notably, since these deviations are determined at the individual subject level, they can allow the deciphering of heterogeneity through stratification,21 and the tailoring of predictions. As such models are defined along the lifespan, they could also offer an intuitive framework for the early identification of risk, and a means to select interventions that help to bring individual-level trajectories closer to the reference range.
Precision psychiatry through advanced deep learning
An area of AI that has recently received a massive amount of attention is that of Large Language Models (LLMs).22,23 LLMs are machine learning models that allow the direct analysis of freely written, unstructured text (or audio recordings), to identify concepts and meaning in such texts, and use them for prediction in clinically relevant contexts. A very promising application of such “Natural Language Processing” (NLP) is the analysis of clinical notes, as these encapsulate the expertise of medical experts and are still routinely generated in clinical practice.
Recent work has demonstrated that LLM-based analysis of such clinical notes reaches superior prediction performance across a variety of prediction tasks, including diagnostic classification.4 Notably, the application of LLMs and other techniques based on the so-called “transformers” also allow the integration across different data modalities. For example, approaches for the combination of imaging data and clinical notes has been successfully applied in the radiology field.3 Such integration could prospectively enable the generation of case reports from data that can be objectively, reproducibly and rapidly acquired, such as brain structural magnetic resonance imaging data. Natural language processing techniques may effectively extend to speech recordings that could be acquired as part of clinical assessments or during psychotherapy, and that may capture clinically-relevant features that are not directly reflected in other data types.
Recent work has demonstrated that LLM-based analysis of such clinical notes reaches superior prediction performance across a variety of prediction tasks, including diagnostic classification.4
Finally, due to the ability of large language models to produce human-like text in response to prompts has fueled interest in their use as chatbots. Such conversational interfaces have been implemented in numerous mental health related applications that cover a variety of mental health indications but frequently lack an empirical evidence base.24 While machine learning models in general face significant obstacles with respect to predictive value, generalizability, and the potential for clinical translation, deep learning and language models in particular still need to overcome fundamental challenges with respect to trustworthiness and safety for tools, such as language models and chatbots to be responsibly implemented in clinical practice.25
While machine learning models in general face significant obstacles with respect to predictive value, generalizability, and the potential for clinical translation, deep learning and language models in particular still need to overcome fundamental challenges with respect to trustworthiness and safety.25
Ethical and legal considerations
Due to the complexity of artificial intelligence methods, the often sensitive nature of data considered for such approaches in psychiatry, as well as the possible vulnerability of affected individuals, there are significant ethical and legal challenges that need to be addressed prior to clinical use of AI-based applications.26 Besides the accuracy and generalizability of the models, these considerations particularly include the transparency by which models arrive at a given prediction, as well as the literacy of involved stakeholders to interpret this model output. Transparency of the models would support the shared decision making between medical experts and patients and thus patient autonomy, and is a core focus of ‘explainable AI’ research.27 This is particularly relevant for machine learning models that have complex architectures, such as deep learning models, and may yield predictions that are not trustworthy.28
The literacy of stakeholders with regards to interpreting the meaning of model predictions in the context in which such predictions are made, data privacy and safety, as well as the appropriate addressing of incidental findings are further important considerations from an ethics and legal perspective.26 Notably, the availability of AI based digital tools has the potential to increase mental healthcare access and thus contribute to health equity.29 However, access to technological infrastructure and digital literacy are involved by several factors, including disability status, that may also lead to an amplification of inequity.30 The careful addressing of these considerations, together with a participatory approach that involves all stakeholders during the development process, will be critical for the ethical and save implementation of AI based tools in clinical practice.
The literacy of stakeholders with regards to interpreting the meaning of model predictions in the context in which such predictions are made, data privacy and safety, as well as the appropriate addressing of incidental findings are further important considerations from an ethics and legal perspective.26
In summary, artificial intelligence offers a highly promising conceptual and methodological framework for advancing personalized psychiatry. Given the increasing availability of high-quality, harmonized data resources that are geared towards AI application, and technological advances for the effective and safe integration of multimodal information, the basis for the discovery of clinically useful models is being rapidly established. It appears likely that this will translate into a substantial increase in AI-focused research in psychiatry, and contribute to the ever-increasing adoption of AI tools in clinical practice.