For decades, scientists have looked for correlates for schizophrenia in the brain. The ultimate hope is that clinicians can use the biological information of a given individual to prevent illness onset and progression, develop new treatments, or use personalise already available treatments.1 This is occurring in other fields, such as cancer,2 but not yet in psychiatry.3
How to develop clinically useful biomarkers
Akin to drug development, biomarker development should also proceed in a series of contingent steps.4
Step one. The first step is the identification of a biological measure scalable to the clinic (Figure 1). For instance, thanks to Positron Emission Tomography (PET) we know that treatment response is most likely in individuals with increased dopamine synthesis capacity in the striatum,5 highlighting striatal function as a target for biomarker development. However, it is unlikely that PET scans will be used routinely in the clinic given the high cost and limited access, whereas Magnetic Resonance Imaging (MRI)6 and electroencephalogram (EEG)7 may be more accessible. Also, as part of this initial step, it is important to address clinical questions for which biological measurements are informative beyond the clinicians’ judgments.
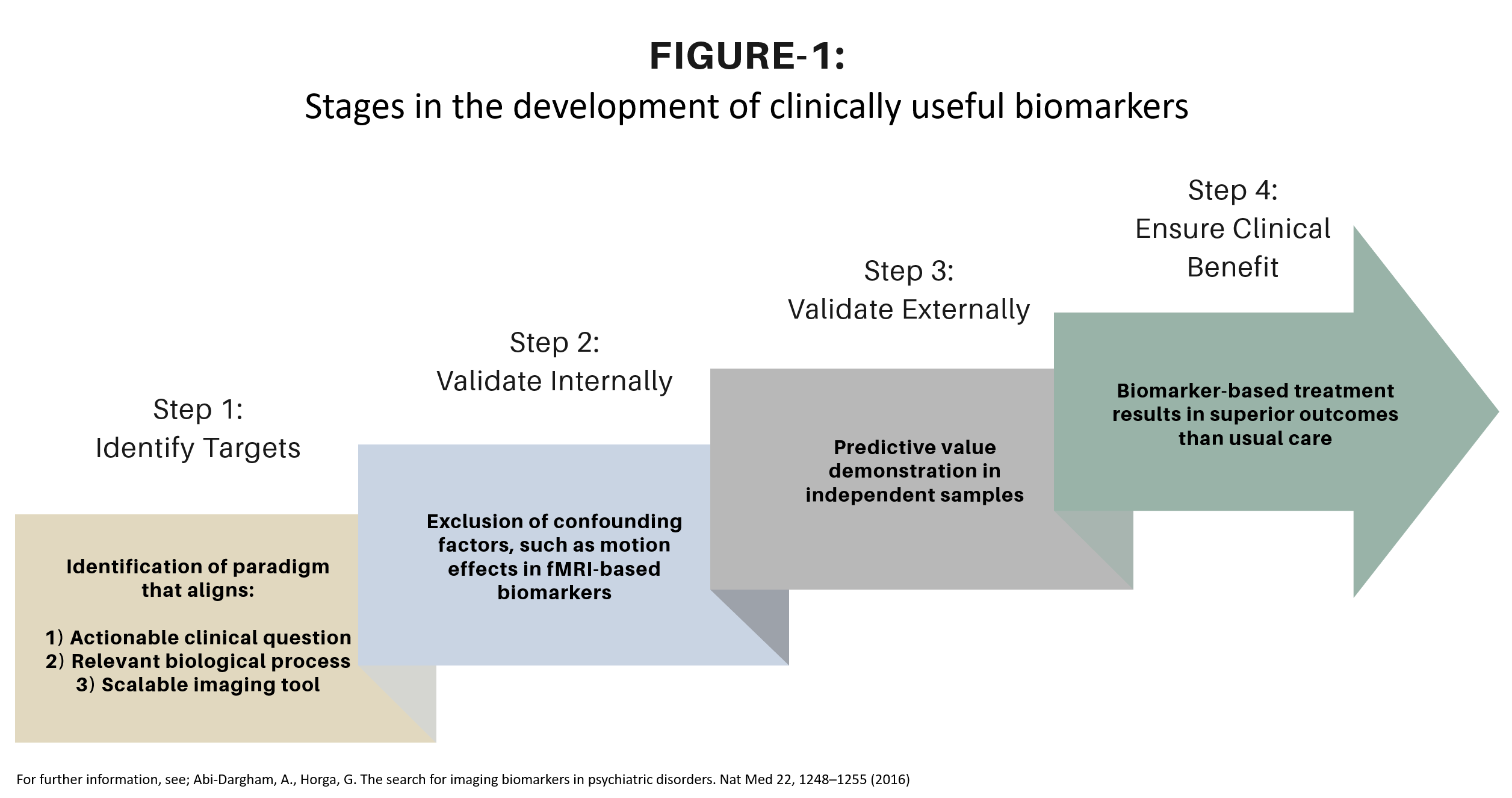
Figure 1. Stages in the development of clinically useful biomarkers
Figure 1. Stages of biomarker discovery from identifying targets (Step 1) to clinical benefit verification (Step 4).4
A biomarker for schizophrenia diagnosis is unlikely to inform clinical decisions, since a diagnostic interview is much easier and is necessary for understanding the symptoms but also the treatment needs for a given individual. However, clinical assessments are limited when it comes to predicting the likelihood of treatment response8 or risk of relapse after discontinuation of treatment.9 Consequently, if biomarkers were ever able to inform these predictions, they could influence clinical decisions. Finally, as part of this initial step, it is important to utilise a potential biological mechanism related to the clinical outcome of interest. For example, there seems to be a robust relationship between striatal function and treatment response in schizophrenia,5 making striatal function a potential target for biomarker development.
There seems to be a robust relationship between striatal function and treatment response in schizophrenia,5 making striatal function a potential target for biomarker development.
Step two. Once there is alignment between an actionable clinical question, a relevant biological process, and a scalable measure, the second step is internal validation. The goal at this stage is to show that the relationship between the biomarker and the clinical phenomena is mediated by the biological phenomena of interest, rather than by confounding factors. For example, there might be a close relationship between a resting-state fMRI biomarker value and treatment response, but this could be because the biomarker is very sensitive to head motion in the scanner and participants with greater motion are more likely to present the outcome of interest. This confounding needs to be addressed in the analyses. In neuroimaging, this often means regressing out nuisance variables (e.g., head motion, signals related to breathing and heart rate, drugs, and so forth).
Step three. If an association is found that cannot, beyond a reasonable doubt, be better explained by confounding factors, the third step is external validation. The goal is to confirm that the associations between the biomarker and clinical outcomes are generalisable and not just a reflection of idiosyncrasies of the data, a phenomenon known as overfitting.10 Importantly, at this stage it is relevant to demonstrate the ability to classify each individual according to the predicted clinical outcome of interest, thus generating sensitivity, specificity and accuracy.11 The APA working group on biomarkers recommends at least 80% accuracy for clinical utility,12 although other authors have suggested that the minimum accuracy for clinical utility may depend on the circumstances (i.e., whether the biomarker is for screening or is confirmatory, the consequences of misclassification, alternative methods to the biomarker etc.), and that lower accuracy levels may be acceptable in some cases.13 For example, predictions of suicidal behaviour at the individual level are not better than chance.14 Nonetheless, even a biomarker with marginal increments in sensitivity that does not have high financial, personal or system costs could still be desirable. This third step is the most challenging and it is here that most biomarkers fail to progress.
Step four. The fourth and final step is the litmus test for demonstrating clinical utility, in a similar way as drugs need to demonstrate effectiveness, in addition to efficacy. This means demonstrating, for instance, that the biomarker improves clinical outcomes cost-effectively. This step would be incomplete if the gap between demonstrated effectiveness and adoption in the ‘real world’ is not bridged by implementation research projects that facilitate overcoming barriers to routine implementation of biomarkers. We should learn from the lessons of long-acting injectables or clozapine in the treatment of schizophrenia, very effective but underused treatments.15 This long and complex pipeline illustrates the challenges associated with bringing biomarkers to the clinic (Figure 1).
When implementing biomarker based care, we will need to apply lessons from other examples of scaling practice innovation in psychiatry.
Current state of schizophrenia biomarkers
In a recent expert review,13 we highlighted the Striatal Connectivity Index (SCI)16 and the Functional Striatal Abnormalities (FSA)17 as the most developed biomarkers for schizophrenia, with promising data on their external validity. Both biomarkers are hypothesis-driven, focusing on striatal function, since this is known to be a likely element in the pathophysiology of schizophrenia.6

Figure 2. Exemplars of clinically useful biomarkers of Schizophrenia
A. Measurement of functional connectivity: Data is collected using resting-state functional magnetic resonance imaging (fMRI). The acquired images reflect the blood oxygen level-dependent (BOLD) signal in space over time. The spontaneous fluctuation of the BOLD signal over time for a given brain region is known as a time series for that region and indicates its neuronal activity over time. The relationship between the timeseries of different brain regions reflects their functional connectivity (I.e., if the signal increases and decreases simultaneously, they are functionally connected). The functional connectivity of regions of interest can be further analyzed (e.g., selecting connections of interest and weighing their functional connectivity strength) to generate individual scores. Exemplars of this approach are the SCI, shown in panel B,16 and the FSA, shown in panel C.17 B. The SCI for a given individual reflects their functional connections in 91 couplets between the striatum and other regions in the brain, which predict treatment response. In the original publication16, its individual value had ~ 80% accuracy in predicting future treatment response. C. The FSA does further processing of functional connectivity (I.e., the striatal fractional amplitude of low-frequency fluctuations (fALFF) analysis, intra-striatal and extra-striatal connectivity) to generate individual scores that can discriminate schizophrenia from healthy controls. In the original publication17, individual FSA scores had an accuracy of ~80% separating schizophrenia from healthy control cases.
Watch Jose M. Rubio explaining the Figure 2. Exemplars of clinically useful biomarkers of Schizophrenia. Find the link to the full video after the article.
Rs-fMRI scans reflect the blood oxygen level dependent (BOLD) signal over time, which is considered an indirect measure of neuronal activity. The BOLD signal in a region of interest (ROI) – ranging from individual voxels to entire anatomical structures – constitutes over time a ‘time series’ for that ROI. These ‘time series’ are analysed in various ways to reflect processes of interest. For example, the synchronicity of the ‘time series’ between ROIs reflects their functional connectivity, whereas analyses such as fractional Amplitude Low Level Fluctuations (fALFF) are reflective of the spontaneous activity of that ROI. The results of these ‘time series’ analyses (e.g., functional connectivity, fALFF etc.) can then be used to select the ‘features’ that will be used to make classifications. In the FSA, features from striatal fALFF and intra- and extrastriatal functional connectivity were used to differentiate cases from controls with >80% accuracy, being specific for schizophrenia (i.e., not being able to classify patients from controls in other conditions such as ADHD or OCD). Alternatively, in the SCI, functional connections of the striatum associated with treatment response were used to predict non-response with 78% accuracy in an independent sample. Predicting who will be non-responsive to treatment could potentially inform the decision on when to try new treatments, saving time and maybe even morbidity.
Outlook
These findings are encouraging and indicate that there is potential value in neuroimaging biomarkers in schizophrenia, although it is important that these results are replicated under a variety of conditions in the future (i.e., other scanner type, sociodemographic characteristics, treatments etc.). Also, we need to understand better the properties of these biomarkers: their test-retest reliability, whether their performance holds across population characteristics or clinical situations, and whether there are MRI imaging parameters that may influence predictive performance or reliability.18 The biomarker development process is dynamic and should be seen as an ever-evolving process. By this I mean that biomarkers are a part of a continuous evolution as parameters are fine-tuned in line with the emergence of technical improvements and expansion of access to data. A very good example is seen in the calculation of polygenic risk scores (PRSs) for schizophrenia. These individual-based values reflect the number of risk alleles for schizophrenia that a given individual carries in their genome.19 Initial PRSs used 108 risk loci to measure the genetic burden for schizophrenia,20 but as sample sizes have increased, genome-wide association studies (GWAS) are now able to inform PRSs using 270 distinct common genetic loci,21 increasing the precision of risk estimates.
There is potential value in neuroimaging biomarkers in schizophrenia.
Similar to fMRI-based biomarkers, as more data becomes available and imaging techniques more sophisticated, biomarkers will continue to undergo fine-tuning to optimise their predictive performance and reliability. However, as in the field of genetics, this will require global collaboration and data sharing.22 The robustness of the performance of the FSA classifying cases vs controls shows that biomarkers trained with large datasets can be very robust. Importantly, we will need to shift from datasets of cases vs controls to cases reflecting the clinical predictions of interest: treatment response, relapse risk, treatment-emergent side effects, etc. The development of these data repositories with relevant clinical information through international consortia will be crucial to fine-tuning fMRI biomarkers to a level that makes them clinically useful.
The biomarker development process is dynamic and should be seen as an ever-evolving process.
In conclusion, biomarker development is a long obstacle race to which the field has devoted vast amounts of resources over the last decades. However, the fact that biomarker development has not yet impacted clinical care in psychiatry is not a sign of futile efforts, but rather reflects the complexity of psychiatric conditions. So far, the field has identified scalable measures that engage biologically relevant processes for actionable clinical questions, with data supporting internal and external validity. Overpromising in the field of neuroimaging has resulted in justified skepticism,23 so we should be careful about the predictions that we make. The framework of staged biomarker development4 allows us to better monitor progress and better estimate how much more work remains to bring biomarkers to the clinic. However, in my opinion the progress so far in this line of research merits optimism.
The progress so far merits optimism.
Watch the full video or select a chapter below.
00:00 – Why do we need biomarkers for Schizophrenia?
01:55 – Why don’t we already have biomarkers for Schizophrenia?
03:25 – What is necessary to bring biomarkers to the psychiatric clinic?
06:19 – What is the current state of developing biomarkers in Schizophrenia?
07:26 – Why are the current biomarkers focusing specifically on the striatial function
08:16 – Development of neuroimaging biomarkers for Schizophrenia. Resting-state fMRI, fALFF, SCI, FSA
12:58 – Why are these biomarkers important?
14:05 – What is next?